The widespread adoption of machine learning has led to its integration in various businesses. Machine learning entails computational data analysis and automated model building.
Since time immemorial, the finance industry has significantly relied on data analysis to execute its functions. However, as time has progressed, manual data analysis has become more tiresome due to the volumes of data. This is where machine learning models come in.
A recent report by Forbes revealed that about 70% of financial service firms have embraced and are using machine learning in one way or the other. Machine learning eased the task of data analysis from finance experts.
However, developing, deploying, and monitoring data models is a difficult task. This is a factor that made many businesses hold back from adopting machine learning in their processes.
Machine learning operations (MLOps) have helped to ease the process of model development and management. MLOps incorporated DevOps and data science to streamline the process of model development and management.
With this, more banks, investment funds, and insurance companies now rely on MLOps to harness insights and gain value from data. MLOps have also helped bridge the gap between the data scientists and the operations personnel in organizations.
Machine learning operations have caused a significant disruption in the normal functioning of the finance industry. In this article, we shall have a look at some interesting facts about MLOps in finance.
1.MLOps Have Increased the Use of Algorithmic Trading
In its early stages, machine learning and artificial intelligence technologies were quickly adopted by hedge fund companies. The hedge fund companies relied on machine learning to automate the purchasing and selling of stocks. The models in use were configured to get triggered when the specified stock prices crossed the defined thresholds.
MLOps have helped hedge funds and other financial institutions build models and trade more effectively. ModelOps integrated machine learning models and artificial intelligence, thereby improving the functionality of models. This increased the use of algorithmic trading methods significantly. Financial institutions could make market predictions and include more variables in their trading systems.
Algorithmic trading has significantly leveraged the use of data models developed using MLOps and managed through ModelOps. ModelOps aims to complement MLOps by offering management for all the developed machine learning models. While MLOps are mostly performed by data scientists, ModelOps involve both the IT and business personnel in an organization.
2. Cybersecurity Is an Integral Use Case for ML
The finance industry was listed as the most targeted industry for cyberattacks in 2020. This, however, is not a shocking deduction because the finance industry had been the top target for 5 consecutive years before that.
In the same year, banking reports depicted that there was a 50% increase in fraud detection rates through the use of new technologies. The adoption rate of machine learning security operations has steadily increased due to its efficiency in reducing cyberattacks.
Machine learning models use historical data to detect anomalous data trends in finance that signify cyberattacks. Machine learning models also identify the attack points.
However, even with the use of machine learning, cybersecurity cases are still hard to detect. This is because the attack methods used by cyberterrorists rapidly change and adapt to new measures set to detect them.
This is where MLOps come in to help in cybersecurity. Cybersecurity professionals continuously analyze the methods used by cyberterrorists. After getting detailed information about the attack methods, the information is sent to machine learning engineers.
Machine learning engineers use MLOps to rapidly develop and deploy predictive models to secure systems against the new attack methods. This helps protect financial systems from vulnerabilities to new attack methods that can be used to infiltrate their systems.
3. Customer Service in Finance Is Reliant on ML
The success of any business in the finance industry is heavily reliant on its customers. Machine learning has been used to improve customer experience. The improvement of customer experience has been done through the deployment of ML or AI-based chatbots.
The chatbots offer 24/7 customer service to ensure that their clients are satisfied. The chatbots are developed using MLOps to integrate machine learning and artificial intelligence. This helps chatbots to mimic natural human conversations when offering necessary help to clients.
4. MLOps Have Improved Risk Management
Machine learning has advanced quickly into many industries. However, this rapid advancement has posed a risk to industries adopting machine learning. The rapid and unregulated development and deployment of models pose a huge model accuracy risk to financial institutions.
Model risks can arise from the invariable use of machine learning models for all business processes. The overreliance on machine learning models causes a risk if incorrect or wrongly designed models are used. Model risks can cause grave consequences to finance institutions. The consequences can include financial losses, poor strategic decisions, damaged reputation, etc.
To ensure such events do not happen, MLOps streamline the process of developing, training, and deploying models. The streamlining of the model creation process helps to avoid any potential risks. This helps to alleviate any potential risks posed by incorrect models.
MLOps streamlined process addresses all possible model error points. The error points addressed are the model design stage, testing stage, implementation, and finally model management.
5. MLOps Offer Scaling Capability
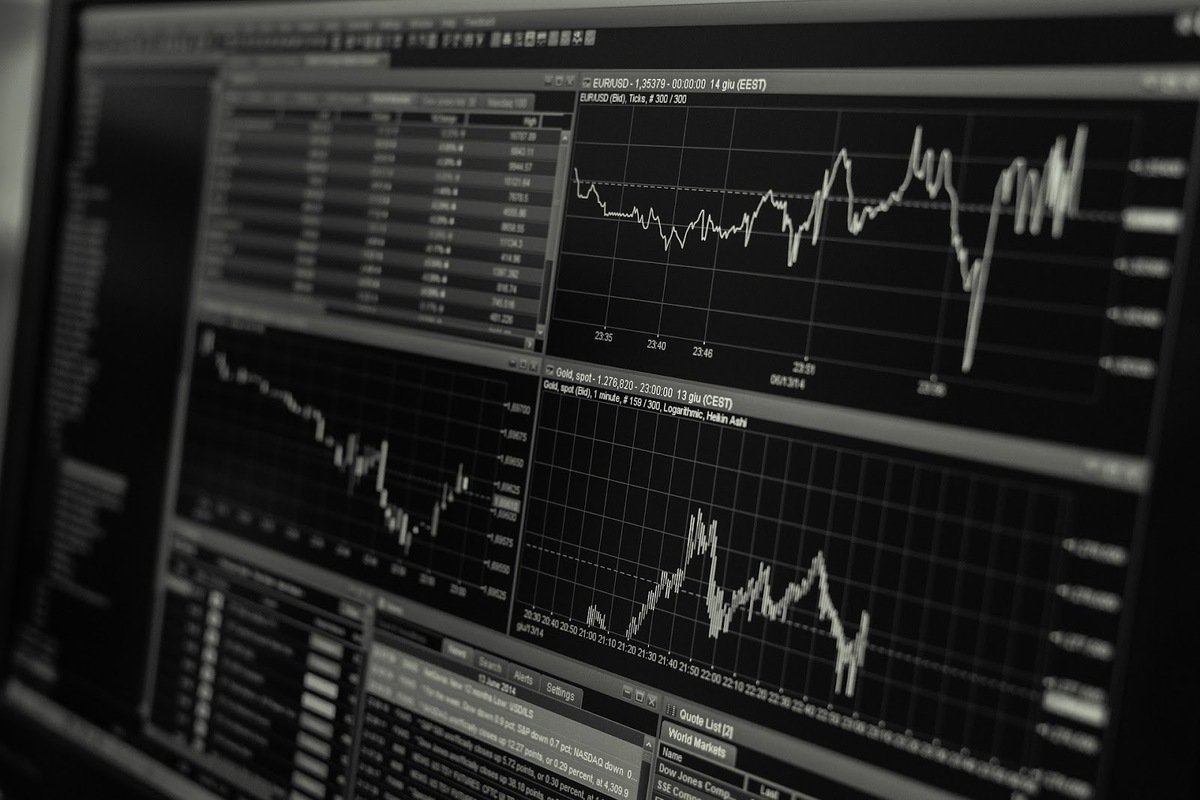
MLOps are an extension of DevOps into the data science field. MLOps rely on industrial-grade tools and utilities. The tools and utilities improve the development and deployment process of machine learning models. The use of industrial-grade tools offers a unique advantage to financial institutions implementing machine learning in their processes.
MLOps help to automate redundant processes in the model development process. Automation of redundant tasks helps businesses to easily integrate new datasets into existing data models to improve accuracy.
Also Read: Dutch Bros Straw Code
Through the use of MLOps, existing models can also be scaled up using deployment technology that supports more use cases or user numbers. The scalability of models is a critical factor to allow the expansion of financial institutions.
MLOps also offer the ability to integrate existing machine learning models with open-source projects like Jupyter notebooks. This creates an avenue for collaboration in the development of machine learning models.
Conclusion
The use of machine learning in the finance industry has offered tremendous improvements and, therefore, better revenues. The future for the finance industry depicts heavy reliance on machine learning and artificial intelligence technologies to improve revenues.
Interesting Reads: Which Item was Invented by a Secretary and Later Sold for $47 Million Dollars?